“The Potential of AI Makes Me Optimistic”
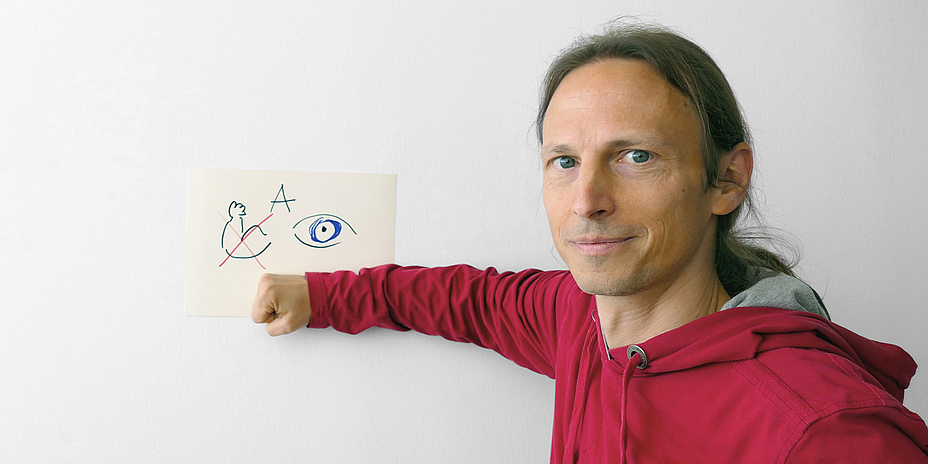
News+Stories: How would you explain to a layperson what artificial intelligence is?
Roman Kern: Historically, in the middle of the last century, people began to think that machines could solve similar tasks as humans. The idea of the well-known Turing test comes from this time. The idea was that you have created an artificial intelligence that is also truly intelligent if you can communicate with it without noticing whether it is a human or a machine. That would be one idea of a definition. Artificial intelligence can be many things in my view. But it is especially relevant and important when it takes on human tasks and can support us. It can be something simple or it can be something complicated – with simple rules or machine learning, deep learning and so on. Therefore, AI is not necessarily defined by its technical realisation, but in terms of what tasks it solves.
What is exciting here is the concept of intelligence. It implies that these systems think for themselves. Something that they do not do, however.
Kern: Exactly. Also, the difference between weak AI and strong AI is important. We are still in the area of weak AI. But the machine cannot yet think or draw conclusions. This can also be seen in modern procedures where the machines “hallucinate” – as we also know from ChatGPT and others. An example of this I like to use is that an AI thinks there is a fire under the water. Physicists will now explain to me that this is possible with certain compounds, but I am simply concerned with things that are illogical. The machines don’t have common sense yet.
Which area of artificial intelligence exactly do you cover in your research?
Kern: Primarily with natural language processing. That is, written text, or more precisely, text written by humans. We communicate a lot in writing and therefore there are many areas of application for AI. For example, search engines use many AI techniques to guess what we are looking for. We then only need to type two words and the search engine knows what we are actually looking for, what the intention behind it is.
What machines are currently very good at is producing text.
Are these, for example, the familiar search suggestions you get when you start typing a search query?
Kern: Exactly. And that is also a nice example of what I meant before. It is not so much the technical realisation, but rather which tasks are solved. Building something like this as a first step is quite simple. It’s just word counting. If someone has typed in New York, then New York City is a good suggestion because many others have typed it in. This is then more or less just dividing two numbers.
What can AIs do now and what can’t they do?
Kern: What machines are currently very good at is producing text. That is, in the simplest scenario, you enter a short text or a few words and the machine figures out how that text would continue. And it turns out that you can solve very many tasks like this. The technical terms for this would be few-shot learning or zero-shot learning. This means that the machine is not trained for certain tasks, but can still solve these tasks. Which brings us back to weak AI and strong AI, which is already such an indicator. A definition of strong AI would also be that one can generalise problem solutions. This is shown by the fact that even if you have not trained the machine to do this, it can still solve these problems.
What exactly is few-shot learning and zero-shot learning?
Kern: With zero-shot learning, a task is solved without the machine having seen the task beforehand, and with few-shot learning, a few examples are enough for the machine to solve the task. Let’s take a translation from German to French: two or three examples are enough for the machine. Here you have a German sentence and a French sentence, and you say this sentence in German is represented by the French sentence.
In the end, it is still humans who devise, build or programme an AI. Is this problematic in some way? Does a certain bias creep in, depending on the application, in that I tell the machine to please take this data or that data or process it this way and not that way?
Kern: This is a big social issue on the one hand and a big technical issue on the other. A technical issue because the machine takes the path of least resistance. This means that if we want to train the AI to distinguish a horse from a zebra, for example, the machine may not learn to distinguish horses from zebras at all, but instead learn to distinguish between grass and steppe. Most zebra photos have a brown background, and most horse photos have a green background. This is a technical challenge, for example – namely, that the machine doesn’t actually learn what you want it to. And the second is the point where the bias, so to speak, or a disruptive factor in training AI arises – that is the social issue. There is the bias in the data itself: bias in, bias out. If I have a data set that already has a certain bias or unfairness in it, then the machine may faithfully replicate that. There are the classic scenarios of job applications where minorities are disadvantaged because until then all minorities have always been disadvantaged and the machine has learned it that way. Or one example from the field of NLP is recognising toxic language. Toxic means insulting or belittling or aggressive or similar. It was found that African American language or African English also tended to be classified in this way on the basis of the training data. That is, even though a person did not express themself toxically, the machine believed that they tended to be toxic anyway. And that is why these people were then disadvantaged.
How biased are we as a society?
Is there a possibility to somehow exclude or get rid of such a bias?
Kern: This needs research. The goal is to train these models in such a way that even if there is a bias in the data sets, you can get this bias out during training. What has become apparent in recent times is that to eliminate such a bias takes a lot of human intervention.
So, to eliminate human prejudices, you need humans again?
Kern: Yes, but the crucial thing is to clearly define a bias. And for that, we as a society must subject ourselves to a reality check: how biased are we as a society? Depending on how I define fairness, I come to different conclusions. And they are even partly mutually exclusive. There is group fairness and there is individual fairness. Group fairness means, for example, that I want to treat a minority equally to the majority. In individual fairness, I would like to treat individuals equally. If someone has the same competences and applies for a job, then they should also get the same opportunities to get the job. And depending on whether I want group fairness or individual fairness, I may not be able to combine both. So, there is no one-hundred-per-cent fair approach.
ChatGPT has sparked the discussion that AI could be used as a ghostwriter for academic or scientific work. Meanwhile, AIs are being developed that are supposed to be able to distinguish between texts written by humans and those automatically generated. Is this a cat-and-mouse game?
Kern: I suspect it might become one. The difficulty is to recognise the automatically generated texts, especially if they are short. But one way it could pan out is to force the manufacturers of these models to require a text to have certain characteristics that you can then check – watermarks, for example. But one shouldn’t assume that only students are using such methods now. Who says that teachers don’t have their teaching materials and also the exam questions generated at the same time?
This interview with Roman Kern is part of the TU Graz dossier "Artificial Intelligence". Find further dossiers in our overview.
There are now also professions where people are thinking about whether AIs can make them redundant or will change them completely. Is this simply a normal sign of technological progress or do you not believe at all that AIs will bring so much change?
Kern: I do assume that a lot will change. There will definitely be certain activities that AI can take over completely, but in the grand scheme of things, I guess our activities will simply change. Especially activities where you write text. For example, when we get an email nowadays, we have to write the answer manually. In the future, it may be enough for me to generate a response by clicking on a button. I only need to check whether I would write it that way. These are, of course, enormous productivity gains that go hand in hand with this. Another example is creativity. You can then get a suggestion for the title of a paper and get inspiration. Another aspect is that we are all now conditioned to use Google search. This could also change to a personalised interaction that allows new types of searches.
What effects can one imagine if the so-called singularity should occur, i.e. the point in time when machines are more intelligent than humans?
Kern: I am optimistic about this because I can see the possibilities and potential. Just by having the dialogue and just having the many biases reflected back that we have as humans, like confirmation bias, etc. Let’s say, for example, that the politicians are provided with an AI as an adviser, which has a different perspective on an issue.
But can you understand people who are afraid of it?
Kern: Yes, of course. It is a loss of control in the end. On the one hand, because in principle people are afraid of any change. In the current situation, where there is a certain amount of uncertainty anyway, you don’t have to stretch your imagination. And people are shaped by films in which we are shown this in a dystopian way – for example Skynet, which wants to destroy us in Terminator.
But a film without dramatic developments would also have a hard time attracting an audience...
Kern: That’s true. A film would be boring without such a drama. And that’s just the problem.
One of my research focuses is on causalities, and machines are very bad at them these days.
Now a change of subject: AI applications like ChatGPT for text or Midjourney for images are now getting a lot of attention. Which lesser-known applications do you find particularly exciting?
Kern: There are lots of them. A Master’s student of mine is looking into ways of giving a voice to a person with speech impairments. The idea is to record words they can pronounce and create a synthetic language from them that can be used to generate any text. This is an application that we are working on right here.
What do you think is the next big innovation or application based on AI?
Kern: That’s a difficult question. One of my research focuses is on causalities, and machines are very bad at them these days. They operate purely on associations, which brings us back again to those photos of zebras and horses. The zebra and the background, in this case the steppe, are associated with each other and belong together for the machine. We do not causally recognise a zebra by its background, but presumably by its stripes – even if we’re not zoologists. I could imagine that the machine could be taught, purely on a technical level, to also take causalities into account. The machine could take the next step: recognising causal relationships and also interpreting them. And the exciting thing is that the machine could then not only recognise cause-and-effect relationships, but also make statements about if-then scenarios. In other words, statements about things that have not yet happened, but that could happen – a kind of future assessment or development assessment. They would then be able to play out scenarios without them having happened.
Would you like to receive the latest stories, news, research stories, interviews or blog posts from TU Graz directly on your smartphone or in your email inbox? Subscribe to the TU Graz Telegram newsletter free of charge.
Kontakt
Roman Kern
Ass.Prof. Dipl.-Ing. Dr.techn.
Institute of Interactive Systems and Data Science
Sandgasse 36/III
8010 Graz
Phone: +43 316 873 30860
rkern @tugraz.at