About Domain Transfer
We introduce a novel learning method for 3D pose estimation from color images. While acquiring annotations for color images is a difficult task, our approach circumvents this problem by learning a mapping from paired color and depth images captured with an RGB-D camera. We jointly learn the pose from synthetic depth images that are easy to generate, and learn to align these synthetic depth images with the real depth images. We show our approach for the task of 3D hand pose estimation and 3D object pose estimation, both from color images only.
This work was supported by the Christian Doppler Laboratory for Semantic 3D Computer Vision, funded in part by Qualcomm Inc.
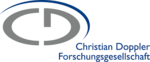
Technical Description
Given a real image of the target object, we first compute the features for the image, map them to the feature space of depth images, and finally use the resulting features as input to another network which predicts the 3D pose. Since this network can be trained very effectively by using synthetic depth images, it performs very well in practice. We demonstrate our approach on the LINEMOD dataset and the STB dataset for 3D object pose estimation and 3D hand pose estimation from color images, respectively.